How can neuroscience support the development of ATM in the future?
- Like
- Digg
- Del
- Tumblr
- VKontakte
- Buffer
- Love This
- Odnoklassniki
- Meneame
- Blogger
- Amazon
- Yahoo Mail
- Gmail
- AOL
- Newsvine
- HackerNews
- Evernote
- MySpace
- Mail.ru
- Viadeo
- Line
- Comments
- Yummly
- SMS
- Viber
- Telegram
- Subscribe
- Skype
- Facebook Messenger
- Kakao
- LiveJournal
- Yammer
- Edgar
- Fintel
- Mix
- Instapaper
- Copy Link
Posted: 6 May 2019 | Paola Tomasello, Stefano Bonelli, Vera Ferraiuolo | No comments yet
As the role of air traffic controllers shifts to a more observatory role, does neuroscience hold the key to ensuring this change doesn’t affect air traffic flows?
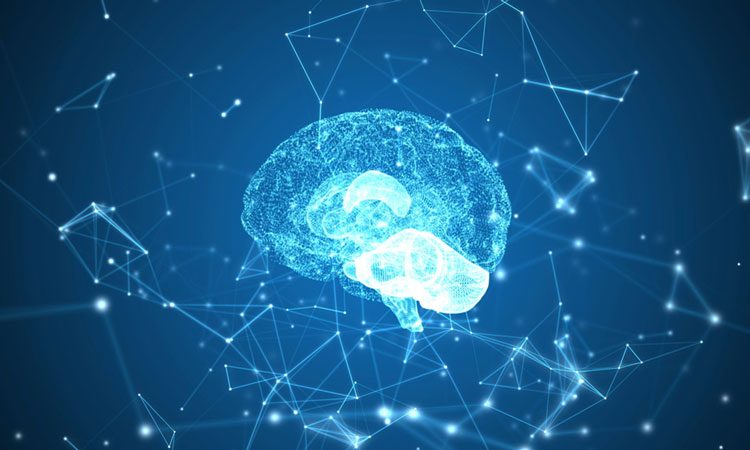
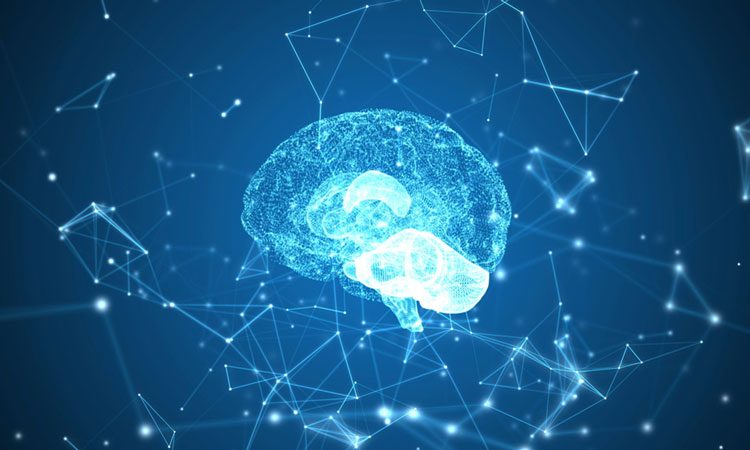
Air traffic is growing as is its complexity. Due to the progressive increase of automation levels, the adoption of innovative concepts such as 4D trajectories, and the introduction of drones into the airspace, experts expect the European air traffic management (ATM) system to face drastic challenges in the nearest future.
Soon, the roles and tasks of controllers will change, and it is vital to enhance the comprehension of human response to such changes. It is also vital to develop tools to investigate aspects like the ability to monitor complex situations and face unexpected disruptions, and to monitor in real time controllers’ fitness to the task, in order to anticipate risks and problems.
The Human Performance Envelope
Several aspects such as stress, workload, attentional resources available, attention focus and so on, impact controllers’ performance. In recent years, the concept of “Human Performance Envelope” (HPE) emerged as a new paradigm in human factors (HFs) to account for this complexity. Rather than focusing on one or two individual factors in isolation (e.g. workload, attention), it considers a range of common factors in accidents and maps how they work in combination to lead to a performance decrement that could affect safety.
It is reasonable to expect that the future air traffic control officer’s (ATCO) HPE will be different from the one we would use today. It will have different underlying HF concepts, or at least a different weight among them. For instance, ATCOs are expected to move to a monitoring position of highly-automated systems, with very few tactical interventions, strategic planning by exception when automation cannot find a solution and the need to intervene rapidly to recover disruptions or unexpected events. As compared to pilots, workload may be even less primary, but with sudden bursts when recovery actions are needed. Indeed, stress will be a major factor both in normal conditions, when ATCOs will need to rely on automation without actively controlling it, and in disruptions. Such a monitoring role will probably require even more attention than pilots exert today. In fact, ATCOs will need to deal with very complex systems, with many interacting elements of different typologies (e.g. RPAS) moving in 4D trajectories across space.
Currently, the main research challenge for complex systems is to explore the HPE in highly-automated environments in an innovative and reliable way. This investigation can provide new knowledge and guidelines needed for designing and implementing higher levels of automation and the related procedures and humans’ roles.
To address this issue Deep Blue, a SME based in Rome and specialist in human factors and safety, coordinated the NINA and STRESS research projects. NINA and STRESS were part of a wider research initiative aimed at investigating the application of neuroscience to the development of new technologies for ATM. In fact, these projects investigated the use of neurophysiological indicators to assess air traffic controllers’ mental state during the execution of operational tasks in highly-automated scenarios. The investigation aimed at deriving guidelines and principles for the design of future ATM systems. The European Commission co-financed both projects in the framework of the SESAR Exploratory Research programme.
Neurometrics at work
Neurophysiological indicators are quite advanced today, offering a unique opportunity to objectively monitor the factors composing the HPE. However, a research gap remains in place concerning the customisation of these indicators to (future) ATM tasks. Both STRESS and NINA aimed to fill this gap. While neurophysiology knows what to monitor to detect stress, what we call stress in ATM may correspond to different patterns of neurological activity as compared to everyday stress. This consideration also applies to other complex HFs concepts like attention and vigilance, which have an everyday meaning and are being studied in contexts different from ATM. In addition, aviation research on neurophysiological indicators has mostly focused on cognitive concepts, traditionally disregarding the stress-related aspects. This oversimplification is hard to justify at the light of current neurophysiological knowledge, where the stress-response has been shown to play a key role in “cognitive” processes like decision-making or attentional focus. A good example is the “startle effect”, defined as an automatic reflex elicited by exposure to a sudden, intense event that violates a pilot’s expectations, and is currently one of the hot topics for pilot performance.
The relevance of stress is also recognised by the EASA, that in the Notice of Proposed Amendment (NPA) addresses the issue of licensing and medical certification of air traffic controllers (EASA, 2012), considering stress and fatigue management as an essential topic for training (AMC1 ATCO.D.045(c)(4) human factors training). In particular, stress demands for a systematic approach. In fact, its importance is likely to grow as systems rely more on automation, and humans move to monitoring positions. A typical case is the automation disruption, when humans have to react quickly in highly-stressful conditions. In these situations, stress is known to influence performance and impair attention, memory and decision-making (Angeli et al., 2004).
In order to capture this level of complexity, STRESS and NINA proposed a multidisciplinary approach. They implemented the high time resolution neurophysiological measurement of air traffic controllers’ stress, workload, attention, cognitive control and vigilance during the execution of operational tasks, within a simulated air traffic control environment reproducing the complexity of future airspace scenarios and associated highly-automated technologies. To achieve this, they carried out data fusion of the following measures: Neural patterns of brain activation (EEG), physiologic indicators (heart activity, galvanic skin response), kinematics (body posture data like joint angles, segment kinematics, segment global positions, body centre of mass) and eye tracking.
The composition of the projects Consortia engaged in these projects reflected such multidisciplinarity, bringing together partners with different expertise. Their competence profiles include a strong understanding of human factors (Deep Blue), a solid experience in the use of neurophysiologic measurements (Sapienza University), a deep knowledge of air traffic management domain (ENAC and Anadolu University), and an overall view on what is the strategic agenda for the development of this domain in the upcoming years (EUROCONTROL).
The STRESS and NINA results represent three possible ways in which neuroscience can support the design and implementation of future ATM systems:
- The neurometrics toolbox for ATM, a tool for the real-time measurement of the controllers’ HPE
- The guidelines for automated systems design, derived from the results of the projects’ experiments
- The proof of concept for adaptive automation linked to real-time measurement of the HPE.
The Neurometrics Toolbox for ATM
The Neurometrics Toolbox for ATM (Figure 1) is able to assess, also in real-time, relevant human factors affecting controllers’ on-the-job performance in terms of neurophysiological variations. In particular, the toolbox can gather different neurophysiological signals and depending on the investigated mental states combine the proper signals and provide an objective measure of the mental states while dealing with the tasks.
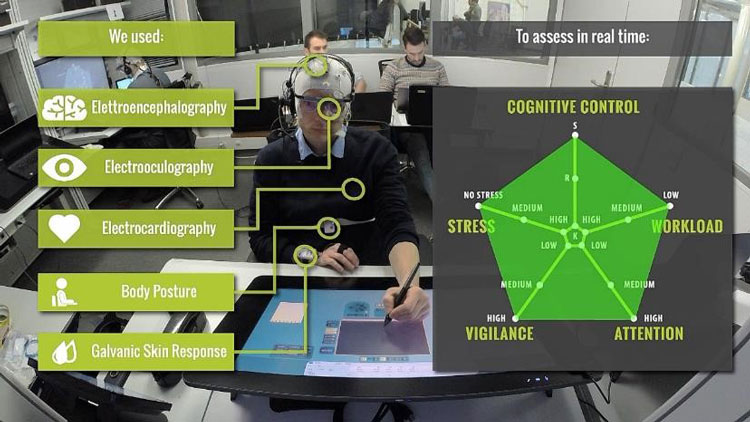
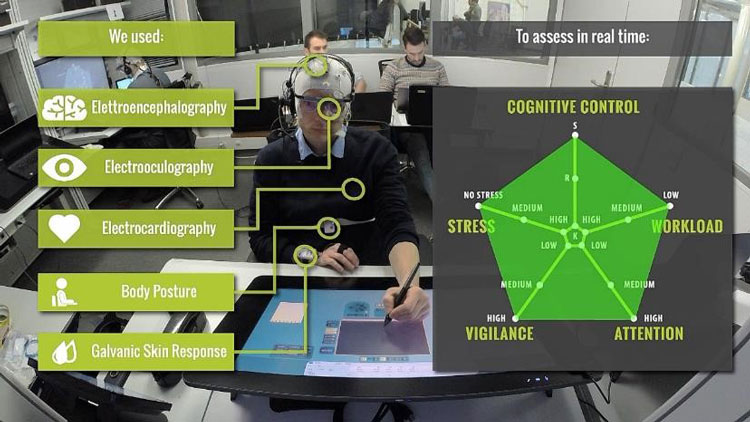
Figure 1 – The concept of the neurometrics toolbox for ATM
The toolbox has been validated in realistic settings and it is very promising in terms of being applied to research activities, automation design and controllers’ training. The Neurometrics Toolbox‘s most obvious objective is therefore to be a human factors tool to be used in research activities and pre-operational validations. In research, it gives the possibility to study the effects of whatever new concepts (e.g. a tool, a working environment, a procedure) on controllers’ HPE, understanding its impact on workload, stress, attention, vigilance and cognitive control on tasks. It is possible to enrich the list of human factors to measure using the same methodology applied in the STRESS project. In more applicative contexts, the Neurometrics Toolbox can be used to assess system changes and understand their impact on controllers. For example, the tool can perform the pre-operational validation of tools and procedures (e.g. to understand the capacity of a sector taking into consideration the impact of traffic on controller’s workload, or to find the better sector configuration in terms of impact on controllers’ stress and workload).
The guidelines for automated systems design
In the framework of the STRESS experiments, we used the toolbox to assess the impact of future highly-automated systems for ATM on controllers’ HPE in order to develop guidelines for the design of such automated systems. Even if they refer to the specific automated systems tested during the project and how the controllers reacted to them, these guidelines could also be generalised to other automated systems. The results showed that training and trust are key aspects when dealing with the design and implementation of highly automated ATM systems, as follows.
The importance of trust
The results of the STRESS experiments demonstrated that the ATCOs who trusted the automation and relied on it had different reactions from the ones not 100 per cent conveying in the new tools. For example, the ones with more trust had the best benefits in terms of workload reduction, while the not-trusting ones were using resources to check if the automation was really behaving as expected. The aspect of trust should be taken into consideration when introducing new automated tools, and this is particularly true for high-level automation. The personnel’s training for automation should always include a detailed explanation of the logic behind the system and should include a part in which the reliability of the automation in different contexts and conditions is proved.
The importance of training
The results of the STRESS experiments demonstrated that the level of confidence with the new HMI and automation has a direct impact on workload, stress and performance. In other words, the impact of automation on HPE can be fully beneficial only if the ATCO is properly trained and has acquired confidence in the use of the new tools. In this regard, high-level automations are quite different from low-level ones, for which the controller is in control. In the latter case, it is easier to check when the controller is able to use the tool. With high automation, in which the role of the controller shifts towards monitoring and supervising, proper confidence and training are harder to detect. The training for highly-automated systems should consider this change in role and include ways of checking if the proper level of proficiency and confidence is reached, even in absence of easy-to-detect external clues (e.g. actions of the controller on the HMI).
The proof-of-concept for adaptive automation
The NINA project has investigated the use of neurophysiological indexes to trigger adaptive automation. The project developed a proof-of-concept for an advanced system able to understand in real time the operator’s psycho-physical state, to match it with the situation in which they are operating and to provide the best automated support accordingly. The NINA system provides real-time monitoring, checking whether the controller’s mental workload, stress, attention, vigilance and other relevant factors are within the safe margins inside the optimal HPE. At the same time, the system is able to detect and react to three different sources of disruption: Traffic complexity, time pressure, and reduced fitness due to increase of controller’s fatigue. When any factor of the HPE exceeds the optimal limit of human performance, the system is able to detect the effect on the controller’s ability to understand the situation and make the right decision in time. Adaptive automation kicks in: The system selects the appropriate supporting tool to be provided to the controller and activates it in order to bring the degraded factors back to the optimal state. Once the optimal condition has been restored, the system deactivates the automated solution and goes back to its normal state. The concept is summarised in Figure 2.
The NINA adaptive automation provides specific support to the four main processes controllers have to perform: Information acquisition, information analysis, decision-making and action implementation. The level of automation of the solutions ranges from low (the system leaves the human completely in charge) to high (the system decides and implements some actions).
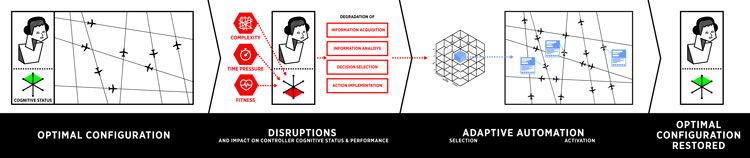
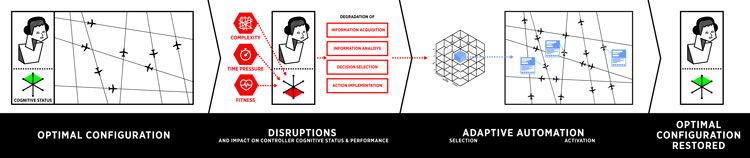
Figure 2: Adaptive automation based on neurophysiological HPE Assessment
Conclusions
The latest research on automation and its impact on controllers cognitive and emotional status is a fundamental pillar for the design and implementation of a safe and efficient future ATM system. The neurophysiological, objective and detailed measurement of controllers’ mental state is starting to be mature enough to be used outside the research domain, by ANSPs, technology designers and training academies. As it becomes easier to use and affordable, it starts to be used more, contributing to a better integration between humans and automation.
References
1.SESAR. (2010). SESAR Human Performance Reference Material – Guidance. Project 16.06.05. D06-001.
2.Edwards, T. (2013). HUMAN PERFORMANCE IN AIR TRAFFIC CONTROL. Thesis submitted to the University of Nottingham for the degree of Doctor of Philosophy. Nottingham.
3.EASA. (2012). AMC/GM to Part-ATCO, Part-ATCO.AR and Part-ATCO.OR.
4.Angeli A et al. (2004). The overtraining syndrome in athletes: a stress-related disorder. J Endocrinol Invest;27, 603-12.
Biography
Related topics
Air traffic control/management (ATC/ATM), Airport Collaborative Decision Making (A-CDM), Regulation and Legislation, Safety, Security