Speech recognition technology for air traffic controllers
- Like
- Digg
- Del
- Tumblr
- VKontakte
- Buffer
- Love This
- Odnoklassniki
- Meneame
- Blogger
- Amazon
- Yahoo Mail
- Gmail
- AOL
- Newsvine
- HackerNews
- Evernote
- MySpace
- Mail.ru
- Viadeo
- Line
- Comments
- Yummly
- SMS
- Viber
- Telegram
- Subscribe
- Skype
- Facebook Messenger
- Kakao
- LiveJournal
- Yammer
- Edgar
- Fintel
- Mix
- Instapaper
- Copy Link
Posted: 26 September 2018 | International Airport Review | No comments yet
Voice recognition that turns speech to text is thought to be the answer to the exponential growth in air traffic ensuring ATC systems are safe and efficient while being up-to-date.
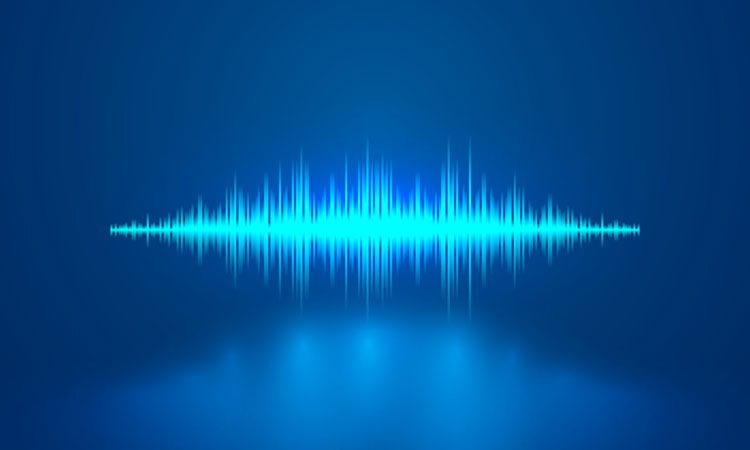
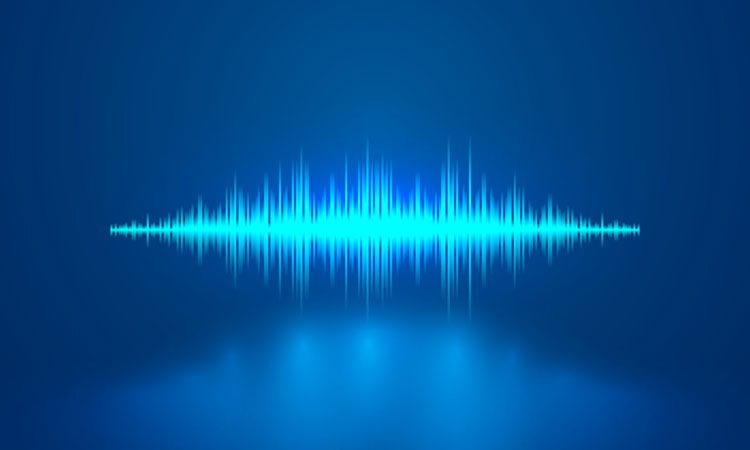
The popularity of air transport continues to grow, placing an even greater workload on air traffic controllers (ATCOs). It has been suggested that their predicament can be improved through an automatic speech recognition system closely integrated with an arrival manager developed by EU and SESAR-funded researchers.
One of the greatest hurdles to introducing higher levels of automation in air traffic management (ATM) is the intensive use of voice radio communication to convey air traffic control (ATC) instructions to pilots. Automatic speech recognition, which converts human speech into texts, can provide a solution to significantly reduce ATCOs workloads and increase ATM efficiency.
The Horizon 2020-funded MALORCA project aimed to reduce the development and maintenance costs of assistant-based speech recognition (ABSR) by using machine learning instead of manual software programming.This initiative was funded within the framework of the SESAR Joint Undertaking, a public-private partnership set up to modernise Europe’s ATM system.
Modern ATC systems must be safe and efficient while being up-to-date. They therefore require significant input from ATCOs, which is currently captured through keyboard and mouse devices. Modern technologies like Air-Ground data link, which in some instances can replace voice communication, will require even more input from ATCOs.
The immense workload of the ATCO can be reduced by ABSR. Project coordinator Hartmut Helmke said: “Fortunately, automatic speech recognition has reached a level of reliability that is sufficient for implementation into an ATM system. However, we need to reduce the transfer costs of speech recognition systems from one approach area to an other one”.
Currently, several speech recognition modules require a manual adaptation to local needs caused by acoustic and language variabilities such as regional accents, phraseology deviations and local constraints. MALORCA proposed a general, cheap and effective solution to automate this re-learning, adaptation and customisation process. This involves automatically learning local speech recognition and ATCO models from radar and speech data recordings.
MALORCA developed new machine learning tools to automatically learn ATCO behaviour and adapt speech recognition models from data recorded by the Air Navigations Service Providers. Machine learning employs statistical techniques that enable computer systems to ‘learn’ and improve their performance on specific tasks over time by exploiting this data, without being explicitly programmed. This will replace much of the manual effort previously required and reduce costs as machine learning of ABSR models makes adaptation to different airports and maintenance cheaper and faster.
Project partners used the output of a so-called ‘Arrival Manager’ for Prague and Vienna airports to automatically split the untranscribed training data into positive and negative chunks through specific confidence metrics. This metric was then used in the developed machine learning algorithms to reinforce learning from adaptation data.
In the context of ABSR the Arrival Manager supports the prediction of the ATC commands that are relevant to the current situation. The predicted commands are compared with the output of the speech recogniser. If a command is not predicted it is assumed that speech recognition system has output a wrong command.
The project thus provides the aviation industry with a practical approach for developing and deploying a state-of-the art speech recognition system and integrating it into today’s voice communication systems for air navigation service providers.
The use of machine learning for speech recognition is only the first test case for its wider application in ATM. Its application in ATM can also help to reduce the adaptation and maintenance of other ATM tools. The adaptation for example of a generic Arrival Manager to specific airports could be the next step.
Related topics
Air traffic control/management (ATC/ATM), New technologies, Passenger volumes, Regulation and Legislation
Related airports
Prague Václav Havel Airport (PRG), Vienna International Airport (VIE)